Google Search Volumes and Economic Activity
September 3rd, 2009 | Published in Uncategorized, Google Finance
We are really excited to launch Google Domestic Trends on Google Finance. This launch was inspired by Hal Varian, our chief economist's research on using Google Trends data to predict economic indicators.
Google Domestic Trends tracks Google search traffic across specific sectors of the economy. The changes in the search volume of a given sector on google.com may provide useful economic insight. We have created 23 indexes that track the major economic sectors, such as retail, auto and unemployment. Each index value is baselined at 1.0 on January 1, 2004 and is calculated and displayed on the Google Finance charts as a 7-day moving average. You can easily compare actual stocks and market indexes to these Google Trends on the charts.
For example, the Google Unemployment Index tracks queries like [unemployment], [social security], [unemployment benefits] and so forth. We see that as the recession took hold in the latter half of 2008, this index increased quite dramatically.
Predicting real-world statistics
We wondered if Google search volumes in a month can help better predict real world statistics well before they are officially tabulated and released.
Predicting retail sales
The US Census Bureau releases the Advance Monthly Retail Sales survey 1–2 weeks after the close of each month. These figures are based on a mail survey from a number of retail establishments and are thought to be useful leading indicators of macroeconomic performance. Learn more about the survey and the procedures followed in constructing these numbers.
The retail sales data is organized according to the NAICS retail trade categories. The data is reported in both seasonally adjusted and unadjusted form; for the analysis in this section, we use only the unadjusted data for the NAICS category 448 (clothing and clothing accessories stores).
Google Domestic Trends data
The Google Retail Index tracks query volumes on Google.com related to the retail trade category. Sample queries are [retail], [bags], [retail sales] and so forth. For this analysis we use the index value (30 day moving average) in the middle of the month. Thus, to predict retail sales of March, 2008 we use the index value on March 15th, 2008.
We observe that the Mean Absolute Error improves from 6.52% to 3.65% (an improvement of 44%)!
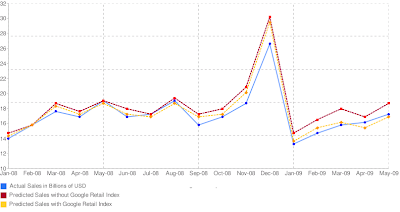
You can read more about Hal's research on using Google Trends data to predict economic activities on the Google Research Blog.
The data for these indexes are available for download — so you can use it with your own models. If you find interesting patterns and uses, feel free to share with us!